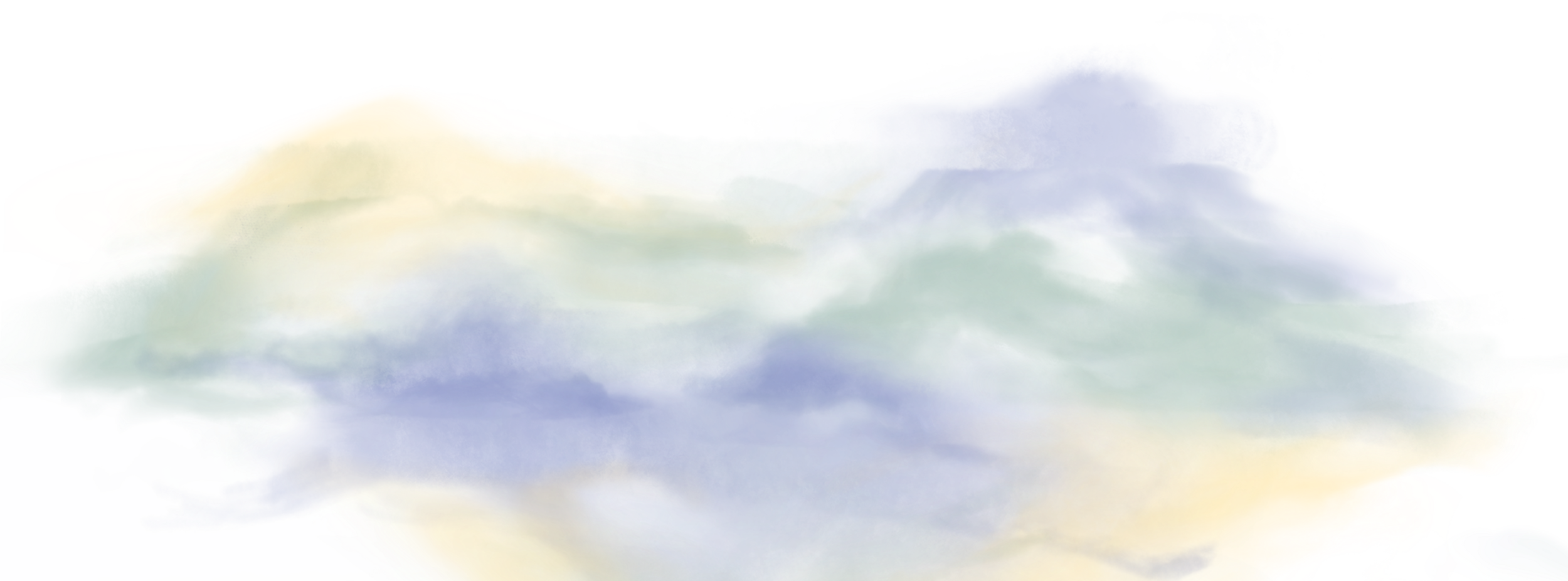
RESEARCH
Advancing the understanding, prediction, and prevention of suicide, nonsuicidal self-injury, and eating disorders.
Our work is inspired by (at least) three principles.
First, psychopathology is inherently individual.
The ways that we think, feel, behave, and interact with others are deeply personal — and influenced by changing social, environmental, and structural contexts. This complex interaction of external factors (e.g., social environment) and internal processes (e.g., emotions, thoughts) gives rise to an individual person’s experience over time, including experiences of mental health and illness. Thus, our lab is dedicated to studying the emergence and persistence of psychopathology from an idiographic (i.e., personalized) perspective. We are specifically interested in understanding why people engage in behaviors that are harmful to themselves, including eating disorder behaviors, nonsuicidal self-injury (NSSI), and suicide.
External and internal processes give rise to people’s experiences of mental health and illness over time.
Second, psychopathology is an immensely complex and dynamic system.
To understand what we mean by a complex system, we can compare complicated vs. complex systems. The behavior of complicated systems emerges from clear cause-and-effect relationships, meaning that we can study complicated systems by breaking them down into their constituent parts and examining each part individually. A car engine is a complicated system: if we combine the same parts in the same way, we will get the same outcome every time — and if the engine breaks down, we can isolate the problem and take clear steps to fix it. In many ways, this is how clinical psychologists have historically approached the problem of studying and treating mental disorders.
However, to understand a complex system, we must study the system as a whole, rather than examining each component in isolation (in other words, the whole is greater than the sum of its parts). This is because complex systems have many components (e.g., emotions, thoughts, environment) with nonlinear interactions and feedback loops, resulting in dynamic adaptation to changing environments and emergent phenomena (behavior that emerges from the interactions among components and cannot be explained by individual components alone). Individual cars are complicated, but traffic patterns are complex.
There are many reasons we believe psychopathology is a complex system, including that it is characterized by multifinality (the same life experiences can cause different mental health outcomes), equifinality (different life experiences can cause the same mental health outcomes), and hysteresis (the state of a system depends on its history).
Our research aims to capture and model the complex dynamics of psychopathology.
We use several methods to study psychopathology as a complex system. For instance, we use ecological momentary assessment with smartphones and wearable biosensors to collect data on mental health symptoms (and their contexts) as they unfold in people’s daily lives in the real world, in real time.
We also build mathematical and computational models to understand individual people’s past and current experiences of mental health and illness, and to predict future outcomes. In the data-driven line of our research, we develop machine learning models for risk prediction using data from smartphones, wearable biosensors, and electronic health records. Our goal is to build models that can accurately predict who is at risk for suicide, NSSI, and eating disorders, and also when individual people are at risk. In the theory-driven line of our work, we formalize theories of psychopathology as mathematical models. We aim to move psychopathology research beyond its reliance on vague verbal theories towards formal theories that require greater specificity and precision, and can be directly observed via computer simulations (see our newest work here formalizing a theory of suicide).
Third, systems of inequality perpetuate and exacerbate experiences of psychopathology.
There are vast disparities and inequities in the development, assessment, and treatment of mental health disorders. As functions of stigma and discrimination, individuals from marginalized backgrounds (along dimensions of race, ethnicity, gender, sexual orientation, and other sociodemographic characteristics) are at increased risk for psychopathology — and receive worse care even when treatment is available.
Therefore, as our lab builds computational models of psychopathology, we also study the causes and consequences of algorithmic bias. Although often heralded as “objective”, algorithms reflect the nature of the data used to train them, and can thus detect, learn, and perpetuate societal injustices. For instance, many healthcare algorithms are less accurate for patients from racial and ethnic minority groups, recidivism algorithms are biased against Black defendants, and large language models can generate toxic and discriminatory text. We are interested in characterizing bias in existing models (e.g., examining whether machine learning model performance is equally accurate across demographic groups), examining the causes of bias in various algorithms (e.g., biases in training data), and studying the impacts of using biased algorithms in clinical settings. Given the many individual, cultural, and systemic biases embedded into psychopathology datasets, we believe it is critical to ensure that our computational models do not perpetuate mental health disparities — but rather help us advance the understanding, prediction, and prevention of suicide and other forms of self-harm for all individuals, towards a more equitable and inclusive mental health landscape.
© Computational Clinical Science Lab. Illustrations designed in partnership with Beyond Bounds Creative.